At SOLVE, we specialize in improving chemical processes, driving both efficiency and sustainability. Through our innovative data collection and machine learning approaches, we deliver flexible future proof solutions for superior results.

Less expensive, low-risk process development.
The data and models we provide for a customer inform which equipment should be purchased, reducing uncertainty and risk in the decision making framework they utilise.
Implementation of more cost efficient and sustainable processes
Our methods enable more efficient and sustainable processes conditions to be utilised by customers, reducing process operating costs and increasing sustainability.
Shorter time-to-market for products
Our data collection is efficient and effective, allowing customers to reduce process development time cycles and produce a scalable efficient process in fewer iterations.
Evidence to support processes to regulators
Our real-world data and models help justify condition choices to regulators easing regulatory burden and demonstrating the trade-offs between different condition choices.
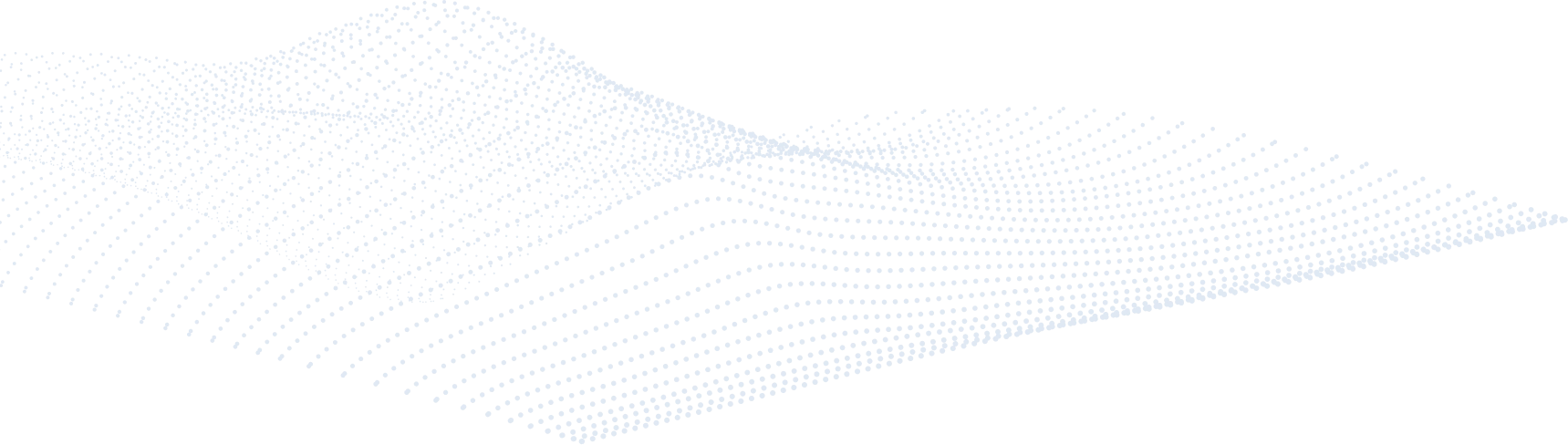
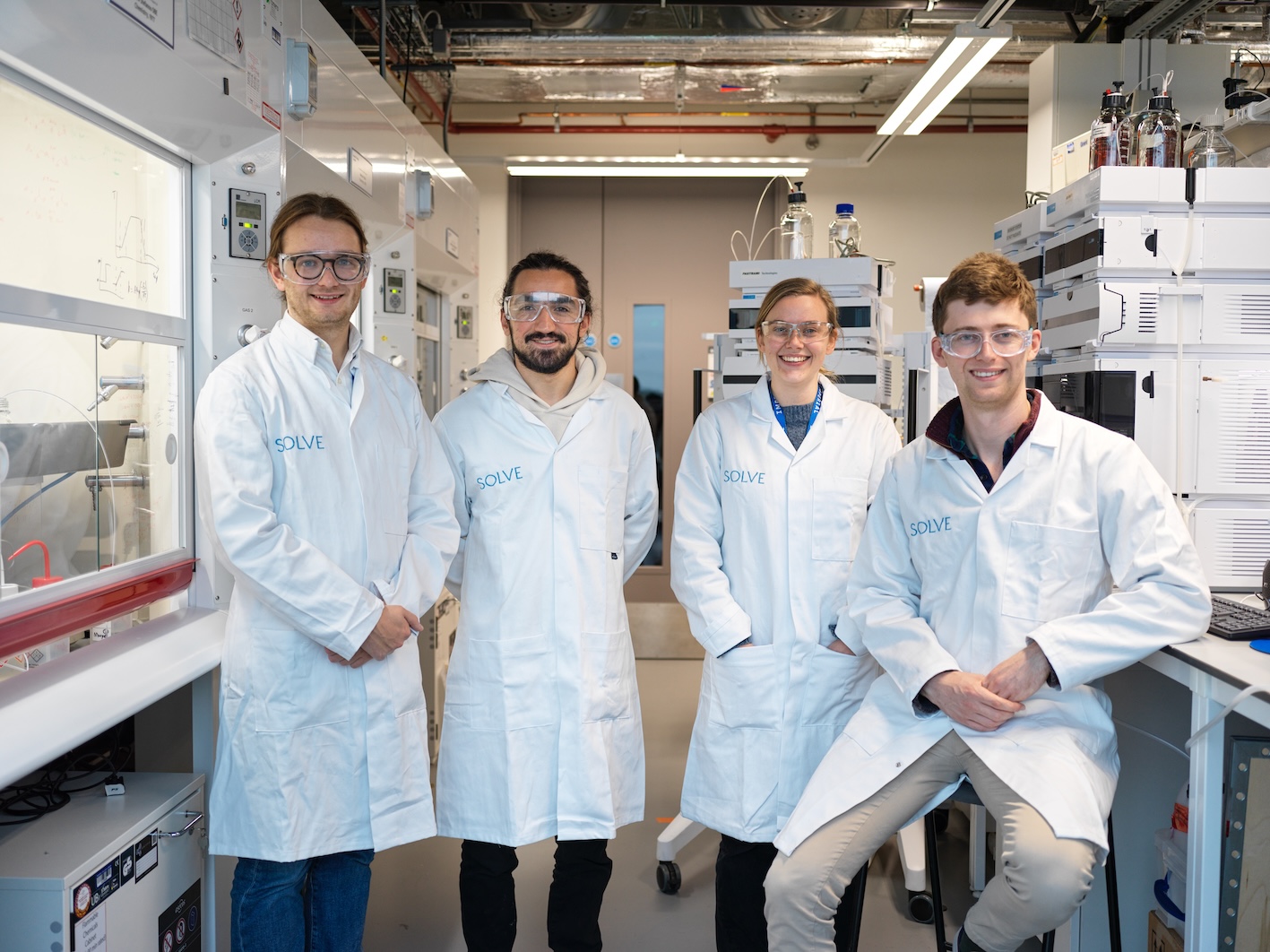
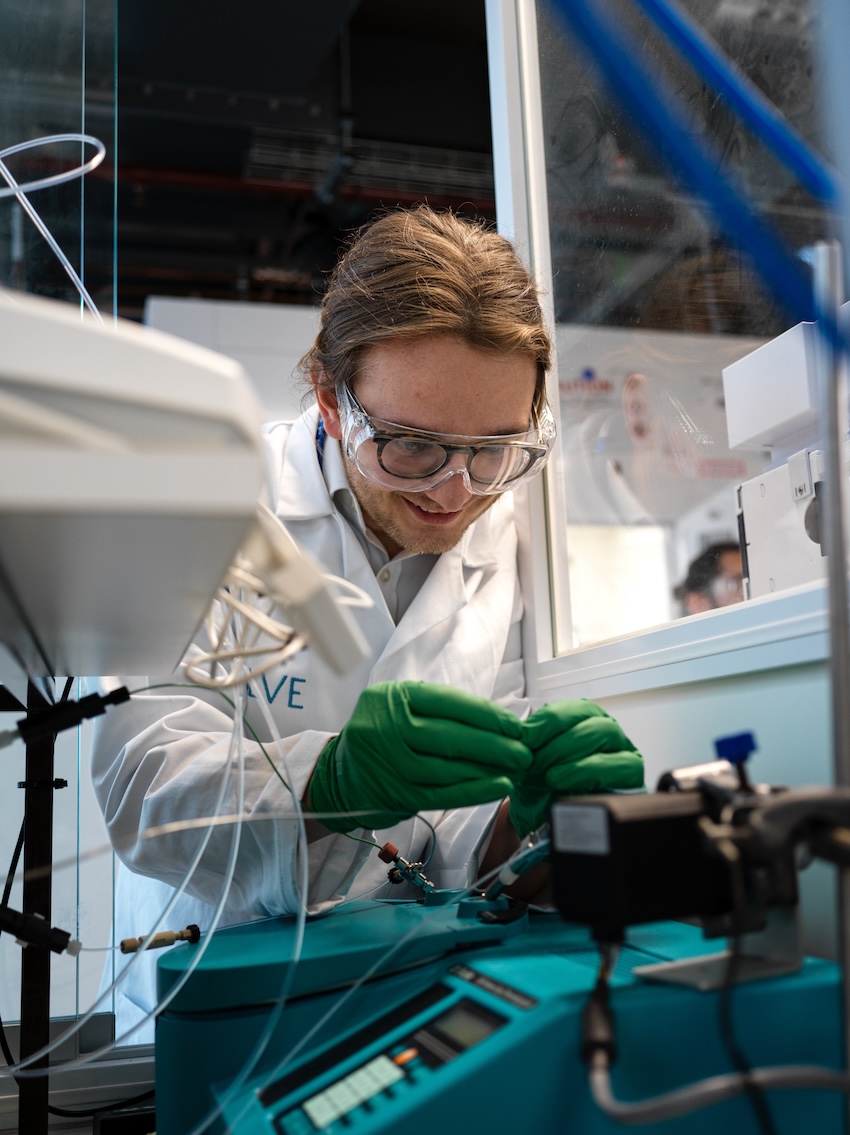
At SOLVE, we are driven by a shared belief that smarter, data-driven solutions can transform the chemical industry and make a real difference in people's lives. Our mission goes beyond simply optimizing processes: we are committed to empowering our clients with flexible, evidence-based options that allow them to apply their expertise in ways that have the greatest impact.
Every member of our team is dedicated to the idea that by improving efficiency and reducing risk, we can help lower the cost of vital products, from pharmaceuticals to agrochemicals, all while driving innovation and setting a new standard for sustainability.